C. Migliorelli, 2017
Methods for noninvasive localization of focal epileptic activity with magnetoencephalography
By Carolina Migliorelli Falcone
Directed by Miguel Angel Mañanas and Joan Francesc Alonso
28.06.2017Summary
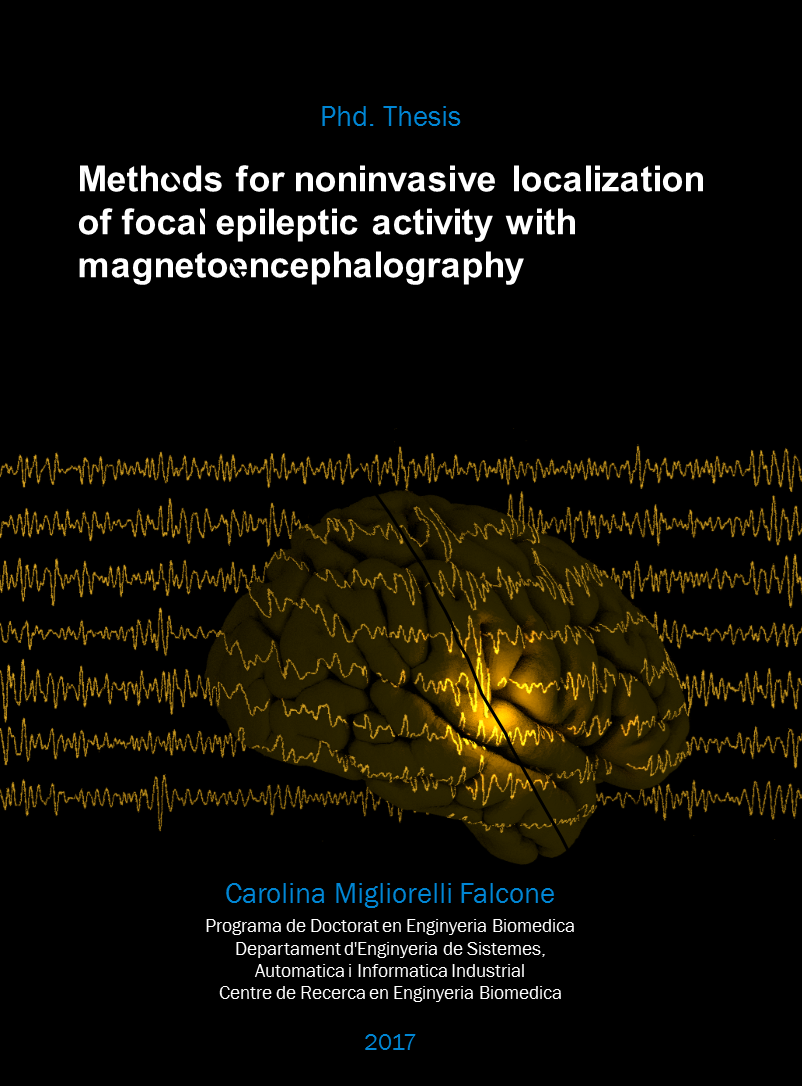
Presurgical evaluation with MEG can guide the placement of intracranial EEG (iEEG), the current gold standard in the clinical practice, and even supply sufficient information for a surgical intervention without invasive recordings, reducing invasiveness, discomfort, and cost of the presurgical epilepsy diagnosis. However, MEG signals have low signal-to-noise ratio compared with iEEG and can sometimes be affected by noise that mask or distort the brain activity. This may prevent the detection of interictal epileptiform discharges (IEDs) and high-frequency oscillations (HFOs), two important biomarkers used in the preoperative evaluation of epilepsy.
In this thesis, the reduction of two kinds of interference is aimed to improve the signal-to-noise ratio of MEG signals: metallic artifacts mask the activity of IEDs; and the high-frequency noise, that mask HFO activity. Considering the large number of MEG channels and the long time of the recordings, reducing noise and marking events manually is a time-consuming task. The algorithms presented in this thesis provide automatic solutions aimed at the reduction of interferences and, the detection of HFOs. This thesis is a compendium composed by the publications of work done during the doctoral period.
Firstly, a novel automatic BSS-based algorithm to reduce metallic interference is presented and validated using simulated and real MEG signals. Three methods are tested: AMUSE, a second-order BSS technique; and INFOMAX and FastICA, based on high-order statistics. The automatic detection algorithm exploits the known characteristics of metallic-related interferences. Results indicate that AMUSE performes better when recovering brain activity and allows an effective removal of artifactual components.
The influence of metallic artifact filtering using the developed algorithm is evaluated in the source localization of IEDs in patients with refractory focal epilepsy. A comparison between the resulting positions of equivalent current dipoles (ECDs) produced by IEDs is performed: without removing metallic interference, rejecting only channels with large metallic artifacts and after BSS-based reduction. The results show that a significant reduction on dispersion is achieved using the BSS-based reduction procedure, yielding feasible locations of ECDs in contrast to the other approaches.
Secondly, an algorithm for the automatic detection of epileptic ripples in MEG using beamformer-based virtual sensors is developed. The automatic detection of ripples is performed using a two-stage approach. In the first step, beamforming is applied to the whole head to determine a region of interest. In the second step, the automatic detection of ripples is performed using the time-frequency characteristics of these oscillations. The performance of the algorithm is evaluated using intracranial EEG recordings as gold standard.
The novel approaches developed in this thesis allow an improved noninvasive detection and localization of interictal epileptic biomarkers, which can help in the delimitation of the epileptogenic zone and guide the placement of intracranial electrodes, or even to determine these areas without additional invasive recordings. As a consequence of this improved detection, and given that interictal biomarkers are much more frequent and easy to record than ictal episodes, the presurgical evaluation process can be more comfortable for the patient and less costly.
Share: